Also,
The Stability-Plasticity Dilemma - is a name used to describe a problem encountered in neural network simulations. Many of these systems, once trained on a given set of exemplar responses, are simply not capable of learning anything new. This prevents the network from being able to
continuously learn while it interacts with its surroundings.
The term is a bit of a misnomer, in that stability-plasticity merely highlights a problem (often incorrectly labeled a dilemma) with conventional artificial neural network learning models. The general behavior of achieving stability and plasticity simultaneously in an adaptive system is not really a dilemma at all. The human brain is a perfect example of a system that quite handily achieves that goal. For that matter, so is the mouse brain. Since it involves asking the question: “How is simultaneous stability and plasticity facilitated within biological learning systems?” perhaps a better label might be, "The Stability-Plasticity Question."
|
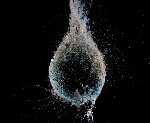 click to enlarge
|
In other words the real crux of the question has been in how to design an
artificial system that—like the mouse brain—is simultaneously sensitive to, but not radically disrupted by, new learning.
This problem manifests in many conventional
ANN models as
catastrophic forgetting. That is, the radical loss of most existing training when an attempt is made to add a single new item to the network's existing (i.e., pre-trained) response repertoire.
. . . . . . .
Enter: Multitemporal Synapses
A new learning method and mechanism, called “
multitemporal synapses” fully solves this problem. In fact, it is capable of providing a considerably greater range of stability-plasticity than is normally associated with human brains.
Like natural neural networks, this method permits the inclusion of both plasticity and stability as two separate, and distinct, components, which act independently of each other. In other words, they are not mutually exclusive. Because of this, multi-temporal synapses are able to
continuously (not merely
continually) learn and adapt, while the network-driven system interacts with its complex environment.
"Don't believe everything
you read on the Internet"
—Abraham Lincoln
| |
. . . . . . .
Problem or Dilemma?
Apparently, there is some disagreement on the Internet, as to whether we should call this a problem, or a dilemma. I recently received an e-mail from someone (May-2013) who insisted it should be called a dilemma, but who would not share who he (or she?) was. His (her?) argument was that everybody agrees with him (or her, whoever he or she is).
There does seem to be some
efferent (“top down”) filtering going on within human perception that causes us to see mutually exclusive choices where no such mutual exclusion actually exists. Consider, for example, the rush in the U.S. to choose between metric and imperial measuring systems back in the
1970s. Because of this added confusion, caused (presumably) by the very nature of how we think, it is probably best to begin with definitions of the terms used.
According to the Oxford-American Dictionary:
dilemma : noun : a situation in which a difficult choice has to be made between two or more alternatives, esp. equally undesirable ones
problem : noun : a matter or situation regarded as unwelcome or harmful and needing to be dealt with and overcome
So, for this to be a dilemma would mean that we are
forced to choose
either one
or the other. That is, if this is a dilemma it is simply
not possible to choose
both. Or, at the very least, we would need to consider a tradeoff between stability and plasticity, where the more we have of either one, the greater would be the negative effect on the other.
While this may have
been the case for
artificial neural networks prior to
multi-temporal synapses, it certainly has not been the case for most biological nervous systems. This demonstrates (rather convincingly) that stability, and plasticity are
NOT mutually exclusive. Nature shows us that we
CAN have both. The traditional limitation was purely an artificial one, caused by the limitations of our existing (artificial) neural network algorithms.
Now, however, with the advent of
multi-temporal synapses, the limitation has been eliminated for artificial neural networks as well. This
problem has been solved — Not by selecting between one
or the other — (as we would have to do if it were an actual dilemma), but by getting rid of the limitation of traditional
artificial network structures. While traditional
ANNs forced us to make the trade-off, we can now include both—and as much of each as we want—in our neural network designs.
Semantically incomprehensible sentences often occur in the literature, when a
solution to the
problem of stability and plasticity is presented, but the word "dilemma" continues to be used. Here are some examples.
***
"Neuronal replicators solve the stability-plasticity dilemma" (title)
***
"Solving the stability-plasticity dilemma in place cells" (title)
***
"The stability-plasticity dilemma must be solved by every brain system that needs to rapidly and adaptively respond to the flood of signals that subserves even the most ordinary experiences." (introduction)
***
[pdf] "How the brain solves this so-called ‘stability–plasticity dilemma’ [1,2] in its sensory areas is largely unresolved." (introduction)
. . . . . . .
Resources
- Consolidation Using Sweep Task Rehearsal: Overcoming the Stability-Plasticity Problem
"This paper extends prior work on knowledge consolidation and the stability-plasticity problem within the context of Lifelong Machine Learning (LML) system. A context-sensitive multiple task learning (csMTL) neural network is used as a consolidated domain knowledge stroe. . ."
- Knowledge Consolidation Using Multiple Task Learning: Overcoming The Stability-Plasticity Problem
"This research focuses on techniques to overcome the stability-plasticity problem in the context of back-propagation neural networks. Our goal is to develop an LML system that retains the knowledge of prior learned tasks without a decrease in the claassification accuracy as new task knowledge is integrated into the CDK net-work."
- Consolidation Using Context-Sensitive Multiple-Task Learning
"An ML3 [Machine Lifelong Learning] system must accurately retain knowledge of prior tasks while consolidating in knowledge of new tasks, overcoming the stability-plasticity problem."
- Methods for reducing interference in the Complementary Learning Systems model: Oscillating inhibition and autonomous memory rehearsal
"The stability–plasticity problem (i.e. how the brain incorporates new information into its model of the world, while at the same time preserving existing knowledge) has been at the forefront of computational memory research for several decades. In this paper, we critically evaluate how well the Complementary Learning Systems theory of hippocampo–cortical interactions addresses the stability–plasticity problem."
- Lifelong Machine Learning Systems: Beyond Learning Algorithms
"Mechanisms that can effectively and efficiently retain learned knowledge over time will suggest new approaches to common knowledge representation. In particular, methods of overcoming the stability-plasticity problem so as to integrate new knowledge into existing knowledge are of value to researchers in AI, cognitive science and neuroscience"
- STOCHASTIC SYNAPTIC PLASTICITY IN DETERMINISTIC aVLSI NETWORKS OF SPIKING NEURONS
"Stochastic learning solves the stability-plasticity problem (Fusi et al., 2000a) but raises new issues related to the generation of the proper noise driving
the synaptic dynamics. . ."
- Critical periods and catastrophic interference effects in the development of self-organising feature maps
"Theories of how the brain acquires knowledge are required to address the stability-plasticity problem, that is, how new knowledge may be incorporated into an information processing system while preserving existing knowledge (Grossberg, 1987). The stability-plasticity problem has particular importance where the individual’s environment is non-stationary – that is, where the information content of experience tends to change over time. "
- Stable Hebbian Learning from Spike Timing-Dependent Plasticity
"These results indicate that stable correlation-based plasticity can be achieved without introducing competition, suggesting that plas-ticity and competition need not coexist in all circuits or at all developmental stages."
- MicroRNA Regulation of the Synaptic Plasticity-Related Gene Arc
"Expression of activity-regulated cytoskeleton associated protein (Arc) is crucial for diverse types of experience-dependent synaptic plasticity and long-term memory in mammals. However, the mechanisms governing Arc-specific translation are little understood. Here, we asked whether Arc translation is regulated by microRNAs."
- Catastrophic Forgetting in Connectionist Networks:
Causes, Consequences and Solutions
"Only rarely (see Box 3) does new learning in natural cognitive systems completely disrupt or erase previously learned information. In other words, natural cognitive systems do not, in general, forget catastrophically. Unfortunately, however, this is precisely what occurs under certain circumstances in distributed connectionist networks." (see: Catastrophic Forgetting)
- Inductive Transfer, Machine Lifelong Learning, and AGI
See: multi-temporal synapses.